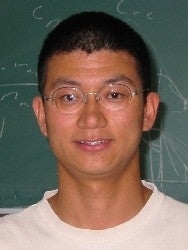
Ph.D., | Texas A&M University, | (2004) |
M.S., | Research Institute of Petroleum Processing, China, | (1999) |
B.S., | Xian Jiao-Tong University, China, | (1996) |
Fault Diagnosis in Batch Chemical Processes
Batch processes are routinely used for manufacturing high value added chemicals such as pharmaceuticals, polymers, and fine chemicals. The batch mode of operation is preferred when the production volume is low and when the materials involved are hard to handle. The operating procedures often follow a golden receipt consisting a series of operating conditions and processing times. Unlike continuous processes, batch processes are characterized by time-varying variables and process parameters. As a result, normal states are not described by the ranges of variables but by a set of acceptable trajectories.
Monitoring tasks in batch processes are very difficult because of many reasons. For example, the absence of steady states operations, high nonlinearities of the processes, lack of online sensors for measuring product composition and the finite duration of the operation are the major problems in developing process supervision systems. Moreover, batch processes are generally operated as multi-product plants where many products share common equipment. Thus the production scheduling must be taken into account for development of supervision systems, because abnormal situations are strongly dependent of the product manufacturing processes. Finally, the variability through batch-to-batch operations due to different operators and initial conditions implies substantial system complexity and uncertainty.
Due to the inherent complexity and flexibility of batch processes, fault diagnosis system that provides fault information to operating and scheduling levels allows one to improve product quality, facilitate active scheduling, and reduce risk of accidents. Fault detection and diagnosis problems have been intensively studied during recent years. Most of the approaches presented so far are applicable to steady state processes. These approaches can be divided into three groups: data driven methods, model based methods and knowledge systems. However, due to the nonlinearity, complexity and absence of steady state operating conditions, the application of these methods to batch processes is usually very difficult. In other words, a successful fault diagnosis scheme for batch processes must be able to handle highly nonlinear and complex process dynamics as well as quickly adapt to batch-to-batch variability of these processes.
Neural Networks Based Approach
The applicability of neural networks for process fault diagnosis has been well demonstrated in recent two decades. However, in real batch processes, due to batch-to-batch variability, operating regions are seldom constant and this may require considerably large amount of re-training of the networks. This reduces the potential of neural network based fault diagnosis systems to apply in batch processes.
In this research, a new neural network based fault diagnosis approach for batch processes is proposed to reduce the time taken to train the neural networks. A feed forward neural network is employed to predict future process output by a series of past process input and output. Past process input/output data are fed into a preprocessor, which fits a second order polynomial to each series of input. Then the coefficients of the polynomials are fed into the network as input. By implementing the input feature extraction process for the neural model, the training time required for the neural process model is drastically reduced with the price of slightly deterioration in model performance. A second neural network realized by a radial basis function (RBF) network is used as the classifier to isolate faults.
To effectively detect sensor faults, newly developed recurrent neural network architecture is utilized to build an accurate process model to generate reliable multi-step-ahead predictions of process output. The difference between process output and model output, namely residual, is obtained and a neural classifier isolates faults by the different structures corresponding to each fault.
Classification Trees Based Approach
To cope with the computational intensity associated with classification tree analysis and the multi-colinearity in the process data, a newly developed process monitoring scheme integrating classification tree and Fisher Discriminant Analysis (FDA) is developed. FDA extracts the most significant components in the original process data and achieves optimal discriminating among different faults. Classification tree uses the FDA scores, which are the lower dimensional representation produced by FDA, to separate observations into different fault classes. A stopping rule is applied to determine the optimal order of FDA. Two case studies are presented to illustrate the effectiveness of the proposed methods compared with the original classification tree. The new method generates better classification accuracy and uses less construction time.
Advanced Safety Instrumented System Design
Safety instrumented system is one of many layers protecting the surroundings from the risks inherent in process facilities. The process control system should keep the process within normal limits. If that does not work, alarms are typically configured to alert the operator to take action, when the process goes beyond the alarm limits, the SIS takes action to shut down the process and contain the hazard. There are usually additional layers of protection in place including mechanical safety devices, relief systems, evacuation procedures, and so on. All the layers must fail before an accident to reach the public.
There have been several guidelines and industrial standards for safety instrumented systems, e.g., Guidelines for Safe Automation of Chemical Processes by AIChEs CCPS; ISA 84.01, and IEC 61508 & 61511. All of these standards are performance oriented, not prescriptive. That is they do not mandate technology, level of redundancy, or manual test intervals. An example is that ANSI/ISA 84.01 defines the reliability requirements for safety interlock systems, but it does not address the dynamics of a process unit to insure that a shutdown will indeed work. Nor does it address the selection of alarm conditions.
While control system providers have been incorporating advanced control technologies, similar sophistication has yet to reach most safety-instrumented systems. A common example is that operators still receive a low flow alarm when s/he stops the corresponding feed pump. Within normal operating range control, process automation has permitted the application of multivariable control schemes. However, SIS, including both alarm and shutdown systems, have simply replicated traditional alarm settings, and multivariable alarm and shutdown systems are rare. Although control system capabilities exist to consider dynamic process modeling, the application of process dynamics to operator alarm conditions has been rare.
A common example of ineffective alarms is the high temperature alarm and shutdown systems for flue gas. Many of these systems have temperature sensors placed too far downstream for operators to react to a rapid temperature rise, resulting in expensive equipment damage. Also start-up of a fired heater/boiler has some traditional interlocks (per NFPA 85) that may not be appropriate unless sufficient information is provided to the operator. In oil/gas separators, sand build-up can impact the dynamics of the alarm and shutdown system. Quantifying these dynamics is critical to maintaining effective abnormal situation management. Another example of the need for multivariable dynamics is the continuous exothermic reactor, such as polymerization reactors. Loss of cooling water typically closes the feed supply valves. Many dynamic water supply conditions or failure of the alarm/shutdown logic solver can result in a reactor runaway.
Dynamic modeling of the reactor, chemical supply, utilities, and control system can provide the operator with early awareness of pending abnormal situations, direct the design of multi-variable alarm/shutdown systems, and help to choose appropriate alarm/shutdown limits. More-complex solutions involving dynamic simulators and fault trees may change the control and operations from reactive to predictive and preventative.
Using the traditional chemical engineering unit operations approach, rather than a chemical process specific approach, the dynamics of the process and the dynamics of the safety instrumented system can be analyzed for defining effective alarm and operator action scenarios to avoid allowing the process to go beyond safety limits.
Lam Research